Sahil Sidheekh
Ph.D. Student,
The University of Texas at Dallas.
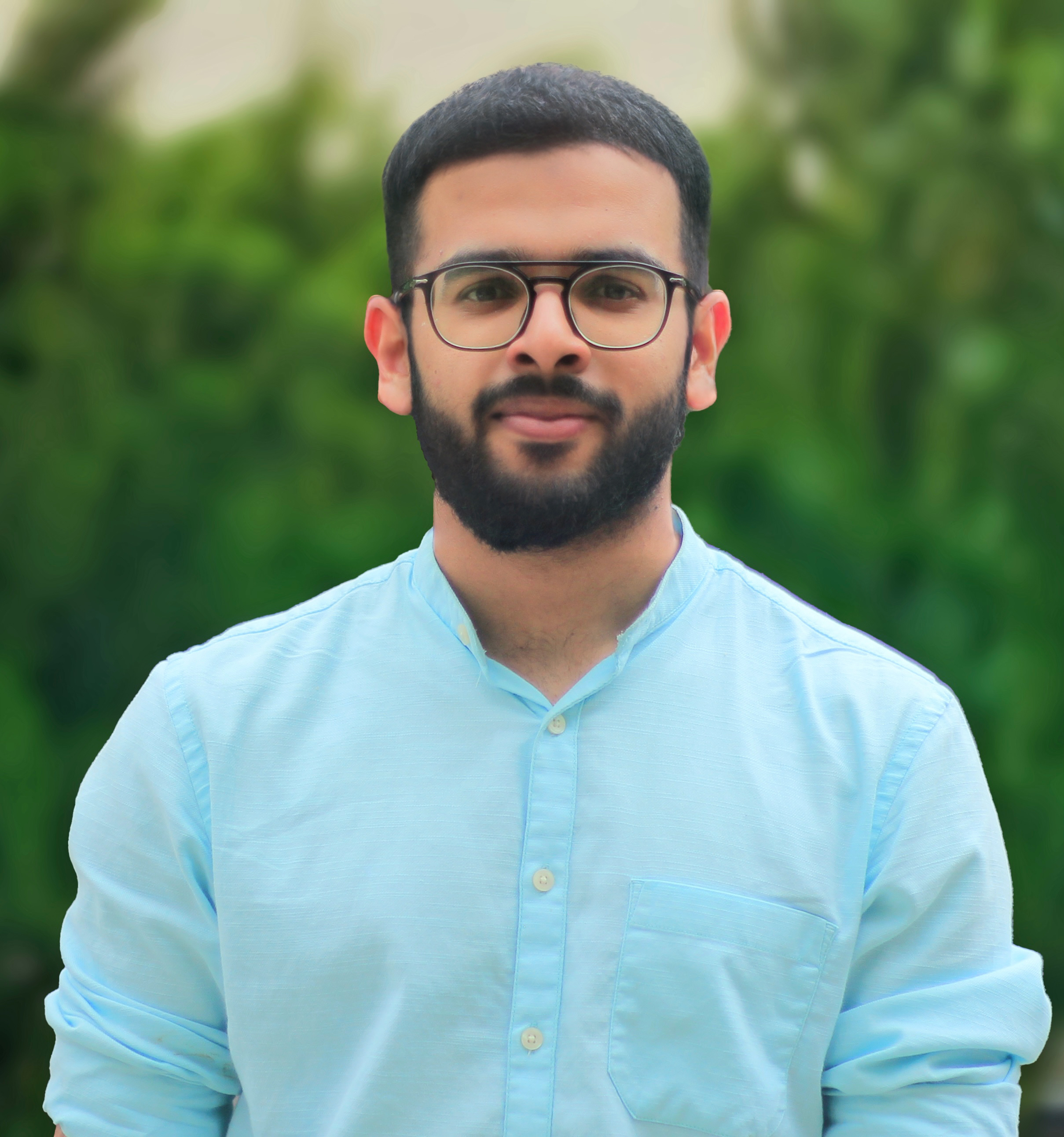
ECSS 3.214, Erik Jonsson School of Engineering & Computer Science, UTD
I am an AI researcher trying to make machines capable of representing knowledge while reasoning about uncertainties in this increasingly complex world.
Presently, I am a Ph.D. student working at the StARLing Lab headed by Dr. Sriraam Natarajan. I work broadly in the domain of Probabilistic Generative Models , Neuro-Symbolic AI, and Meta-learning. I also explore novel applications that can benefit from these reasearch areas, especially problems that can impact society for the better. Previously, I completed by undergraduate studies majoring in Computer Science and Engineering from IIT Ropar, where I was fortunate to be a part of the LSAIML team headed by Dr. Narayanan C. K.
I am passionate about building robust and efficient systems that can reason probabilistically and make interpretable decisions. I enjoy connecting dots and innovating ideas that span multiple disciplines. I have a strong academic background in engineering and machine learning. If you would like to collaborate, please feel free to reach out through email .
News
Jan 4, 2024 | Had an amazing time presenting our tutorial on Deep Tractable Probabilistic Models at CODS-COMADS 2024 in Bangalore! Find the slides here. |
Dec 12, 2023 | Our paper on Credibility Aware Reliable Multi-Modal Fusion Using Probabilistic Circuits has been accepted for presentation at the 2nd Workshop of Deployable AI (DAI), co-located at AAAI 2024. |
Jul 28, 2023 |
Passed my Ph.D. Qualifying Exam! ![]() |
Jul 1, 2023 |
Our paper Bayesian Learning of Probabilistic Circuits with Domain Constraints has been accepted to the 6th Workshop on Tractable Probabilistic Modeling (TPM) at UAI 2023 ![]() |
May 8, 2023 |
Our paper Probabilistic Flow Circuits: Towards Unified Deep Models for Tractable Probabilistic Inference has been accepted for oral presentation at UAI 2023 ![]() |
Apr 20, 2023 |
Organized a 3-day workshop at IIT Madras on Deep Tractable Probabilistic Models, with Prof. Sriraam Natarajan, Saurabh Mathur and Athresh Karanam. Many thanks to Prof. Ravindran and the RBCDSAI team for hosting us. Checkout our github repository for slides/code. |
Mar 20, 2023 |
I will be serving as a workflow chair for AAAI 2024. Happy, Humbled, Honored and Excited !![]() |
Aug 22, 2022 |
I have joined the StARLing Lab at The University of Texas at Dallas as a Ph.D. Student. Excited to learn new things! ![]() |
May 16, 2022 |
Our work VQ-Flows: Vector Quantized Local Normalizing Flows has been accepted to UAI 2022 ![]() |
Jan 19, 2022 |
Our work Machine Learning Methods Trained on Simple Models can Anticipate Crtitical Transitions in Complex Systems has been accepted to the Royal Society Open Science Journal ![]() |
Oct 24, 2021 |
Our work Task Attended Meta-Learning for Few-Shot Learning has been accepted to the NeurIPS 2021 Workshop on Metalearning ![]() |
May 10, 2021 |
Our work On Characterizing GAN Convergence Through Proximal Duality Gap has been accepted to ICML 2021 ![]() |
Apr 10, 2021 |
Our work On Duality Gap as a Measure for Monitoring GAN Training has been accepted to IJCNN 2021 ![]() |
Feb 17, 2021 |
I will be joining Verisk Analytics as an AI/ML Resident. Excited !![]() |
Jan 19, 2021 |
Our work Stress Testing of Meta-learning Approaches for Few-shot Learning has been accepted to the AAAI 2021 Workshop on Meta-learning ![]() |